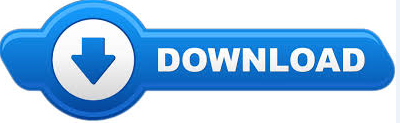
The model is pre-trained using open-source human pose datasets (2) Human-body pose generation based on video streams. We use 2D/3D skeleton point constraints, human height constraints, and generative adversarial network constraints to obtain a more accurate human-body model. The method is divided into two main stages: (1) 3D human pose estimation based on a single frame image. This paper proposes a novel approach to estimate 3D human action via end-to-end learning of deep convolutional neural network to calculate the parameters of the parameterized skinned multi-person linear model.
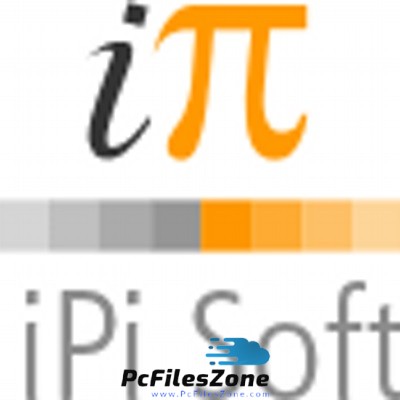
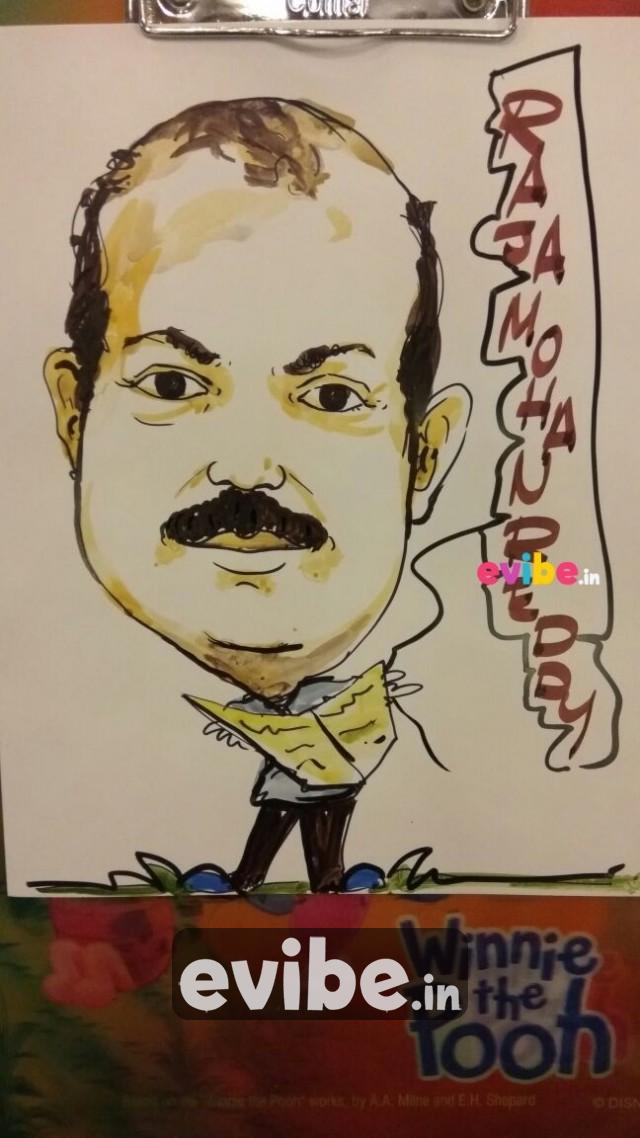
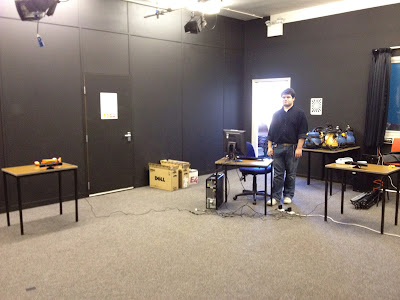
Using video sequences to restore 3D human poses is of great significance in the field of motion capture.
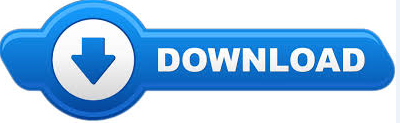